The Transformative Power of Cloud-Based Machine Learning
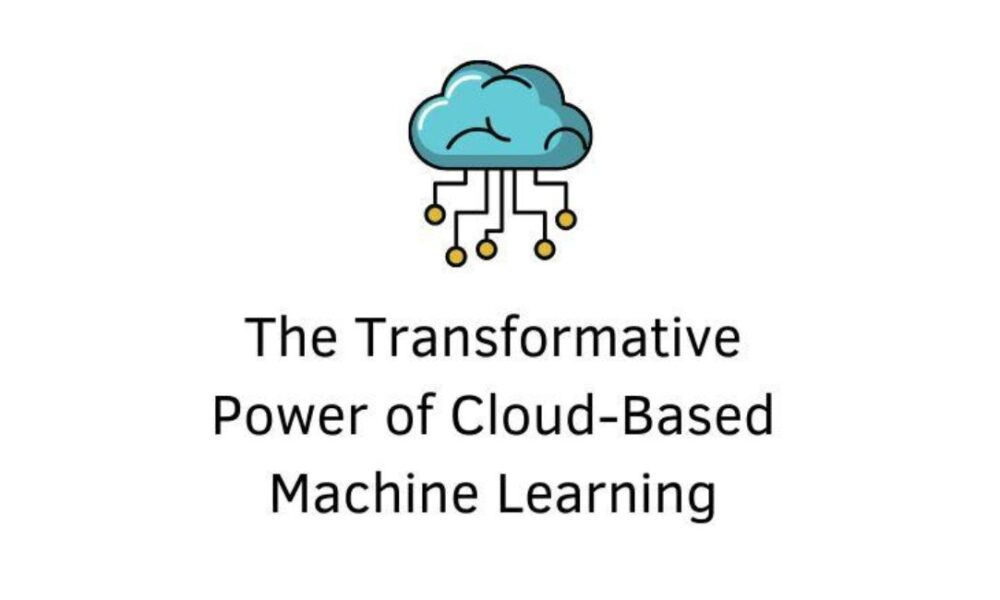
This article delves into transformative innovations in cloud-based machine learning, with Davinder Pal Singh highlighting its impact on scalability, automation, and real-time insights, driving advancements and revolutionizing sectors with cutting-edge solutions and streamlined efficiencies.
Scalable Platforms: The Foundation of Cloud Learning
Cloud-based machine learning thrives on scalable infrastructures that allow organizations to process vast datasets without significant upfront investment. By leveraging on-demand computational power, businesses can perform data-intensive tasks efficiently, unlocking insights that were previously inaccessible. This capability democratizes access to advanced analytics, empowering entities of all sizes to compete on a level playing field.
The flexibility of cloud platforms ensures resources are allocated dynamically, optimizing both cost and performance. This shift toward scalable computing marks a turning point, enabling rapid experimentation and deployment of machine learning models.
Streamlining Processes with Automated Pipelines
Automation in learning pipelines is a cornerstone of cloud-based machine learning. These pipelines seamlessly integrate data collection, preprocessing, model training, and deployment, significantly reducing the need for manual intervention. By automating repetitive tasks, businesses can save time and direct their focus toward refining algorithms and interpreting results.
This innovation accelerates the lifecycle of machine learning projects, allowing organizations to bring solutions to market faster. The ability to iterate quickly on model improvements gives businesses a distinct edge in a competitive environment where time is of the essence.
Integrating Development and Operations with MLOps
Machine Learning Operations (MLOps) frameworks bridge development and production, fostering collaboration across teams to deliver reliable, production-ready machine learning models. By integrating technical and operational workflows, MLOps ensures seamless deployment. Its continuous monitoring and retraining capabilities adapt models to evolving data, maintaining their relevance and accuracy. This adaptability is vital for applications demanding real-time decision-making and frequent updates, making MLOps a cornerstone of effective and scalable machine learning implementation in dynamic environments.
Security and Ethical Challenges in Cloud-Based Learning
As machine learning systems increasingly handle sensitive data, implementing robust security measures has become non-negotiable. Cloud platforms must prioritize advanced encryption protocols, rigorous access controls, and adherence to global compliance standards to protect user information. Data privacy is not merely a technical requirement but a fundamental pillar of trust in these solutions. Equally significant are ethical considerations, as biases in machine learning models and opaque decision-making processes can result in harmful or inequitable outcomes. Adopting transparent, ethical algorithms and fair data practices allows organizations to mitigate risks, foster public confidence, and promote equity. Balancing security and ethics is critical for ensuring sustainable, responsible growth in cloud-based machine learning.
Real-Time Insights: Driving Agile Decision-Making
A key advantage of cloud-based machine learning is its ability to deliver real-time insights by instantly processing data streams. This empowers businesses to respond swiftly to market trends, customer behaviors, and operational challenges, fostering agility and informed decision-making. Real-time analytics is particularly transformative in fast-paced environments like supply chain management and customer service, where rapid response is crucial. By harnessing these insights, organizations can optimize processes, improve user experiences, and fuel innovation, driving sustainable growth and maintaining a competitive edge in dynamic markets.
Unlocking Hidden Patterns with Unsupervised Learning
Unsupervised learning models are pivotal in the cloud ecosystem, analyzing unstructured data to uncover patterns and relationships without labeled datasets. They enable applications like anomaly detection and customer segmentation, optimizing operations and driving strategies. By unlocking hidden opportunities, these evolving models promise transformative breakthroughs in data utilization and organizational insights.
Future Trajectory of Cloud-Based Machine Learning
Cloud-based machine learning continues to evolve, with edge computing emerging as a complementary technology that enhances localized data processing, reduces latency, and improves efficiency. Coupled with advancements in Internet of Things (IoT) devices, this evolution heralds a new era of interconnected, intelligent systems. These innovations promise to seamlessly integrate machine learning into daily operations, establishing it as a core component of modern business strategies. The fusion of AI, IoT, and edge computing unlocks advanced applications, driving transformative outcomes across industries.
In conclusion, Davinder Pal Singh emphasizes that cloud-based machine learning holds transformative potential for innovation and problem-solving. Its success, however, depends on overcoming challenges and fostering collaboration, enabling businesses to adapt, thrive, and lead in an ever-evolving digital landscape.
Source: The Transformative Power of Cloud-Based Machine Learning